Intra-Operative Information: What Surgeons Need, When They Need It
How can we support surgeons by providing them with 3D image information during an operation, so that they know, for instance, where blood vessels are located during a tumor resection? The Creative Unit, composed of scientists from the fields of radiology, computer science, digital media, cognitive systems, and computer graphics, is tackling questions like these. A core challenge is visualizing time-critical 3D information and testing new forms of interaction in the complex operation environment. Live surgical operations demand robust, efficient solutions and visualizations that the operating clinician can perceive and employ immediately. Especially important is that developed systems take operating situations and contexts into account. New methods should be researched that relevant information is automatically delivered to the surgeon at the right point in time.
Liver Segmentation with Deep Learning
Liver segmentation plays an important role when planning surgeries or catheter-based interventions in the liver, and several clinical workflows require a volumetric analysis of the liver. Tumor burden is computed by relating the total volume of tumors found to the liver volume. We use modern Deep Learning approaches to segment the liver and to detect and segment tumors in CT and MR images. Our method is based on an ensemble of U-nets in 2D and 3D.
In this context, we have participated in the LiTS challenge organized in the context of ISBI 2017, and our method scored a good second place (out of 17 participants). Publications are submitted.
Knee Cartilage Analysis
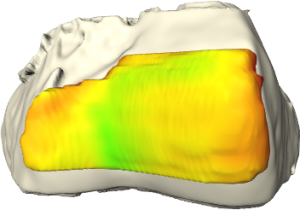
Rendering of cartilage with color-coded thickness in front of Femur bone (patellofemoral joint)
MRI enables non-invasive assessment of articular cartilage, and has long been used for diagnosis of osteoarthritis, one of the most common causes of disabilities. We are working together with researchers from the University Medical Center of Freiburg in order to improve the state of the art both on the imaging and image analysis side, for instance in order to give
- highly precise cartilage thickness maps,
- contact area analysis between opposite cartilages,
- comparative measurements under load (in vivo!), and
- patella instability analysis based on patient-specific movement quantification under load.
Auditory Display for Image-Guided Interventions
During instrument placement using image-guided navigation systems, the clinician must concentrate on a screen. To reduce the clinician’s visual reliance on the screen, this project proposes auditory feedback methods as a stand-alone method or to support visual feedback for placing the navigated medical instrument.
Auditory synthesis models are being developed to augment or replace visual feedback for navigated instrument placement. In contrast to existing approaches which augment but still require a visual display, this method allows view-free needle placement.
Audiovisual feedback shows promising results and establish a basis for applying auditory feedback as a supplement to or replacement of visual information to other navigated interventions for which viewing a patient is beneficial or necessary.
Uncertainty-aware Information Fusion for Soft-tissue Motion Estimation
We devise an uncertainty-aware information fusion algorithm for motion estimation in image-guided soft-tissue intervention and surgery navigation. Soft tissue motion estimation is of great interest in the field of image-guided soft-tissue navigation, because it enables the registration of pre-interventional/pre-operative navigation information on deformable soft-tissue organs. Our proposed algorithm combines various uncertain information sources, such as soft tissue motion measurements, motion dynamics, shape information, etc.
Image Guided Robot control Therapy, Navigation and Intervention
Needle based interventional diagnostics and therapy play nowadays a significant role in minimal invasive medical interventions.
These interventions are usually done manually or based on pre-operative imaging.
However, due to organ movement and tissue-pressure achieving accuracy is still challenging in this area.
In this project, we are trying to reach a better accuracy and more precise targeting of the lesion by integrating a lightweight robot controlled intraoperatively by the user via intraoperative imaging and in an iterative way for a better path-correction.
The high performance and precise algorithms of the image-processing platform MeVisLab is used for viewing, registration of the images and segmentation of the needle or target.
The user can do the path-correction for the needle iteratively, by clicking on the new target of the needle in the intraoperatively shown image; each click can be translated into commands, which are sent to the robot holding and moving the needle.